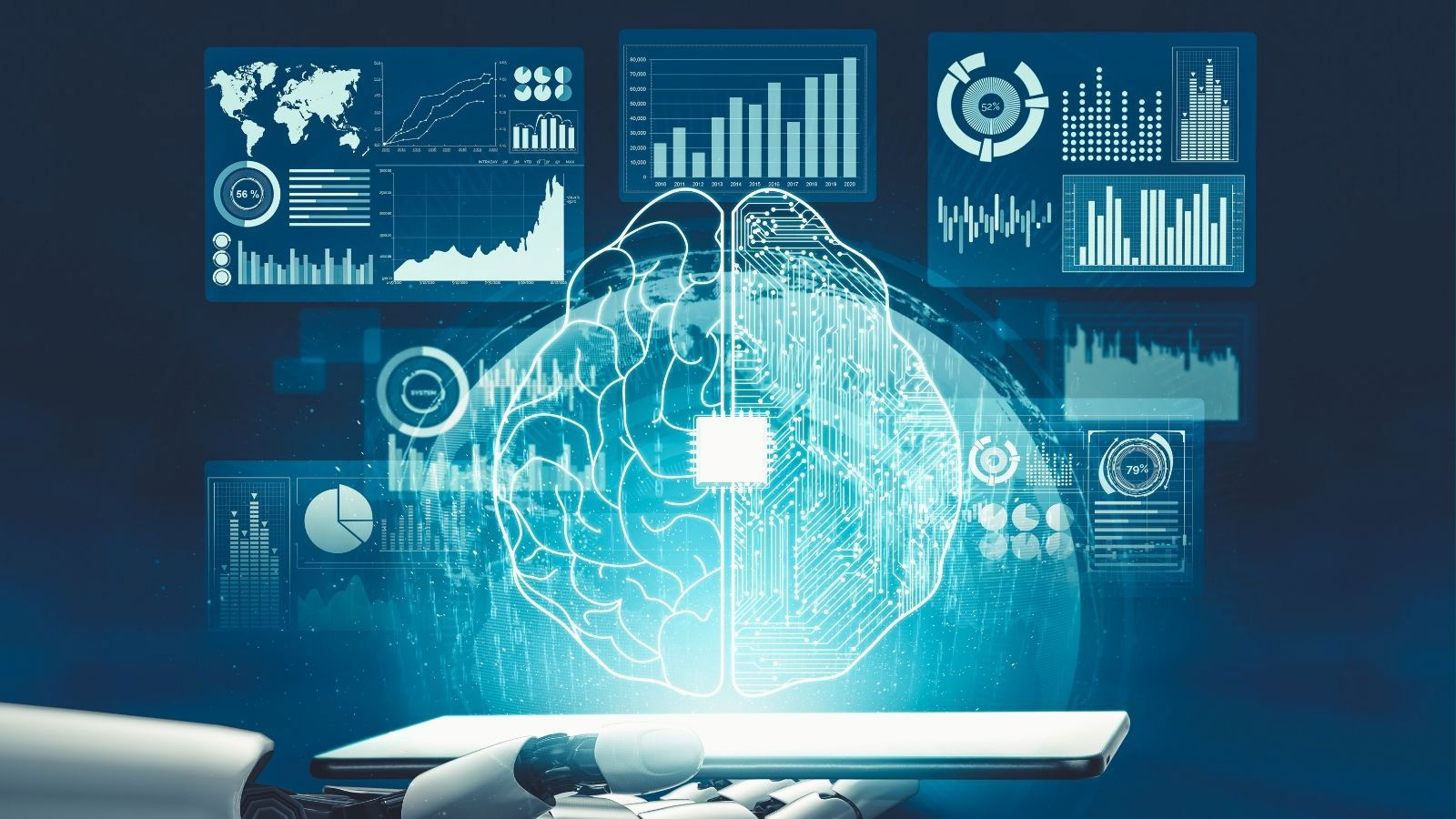
Synthetic data for dummies: 101 on what it is and why marketers should care
share on
As AI ramps up in usage, so do fears surrounding its safety, how it is being trained and where that information is coming from. After all, the information that AI models come up with when you type in a question or ask it to do something comes from somewhere.
That 'somewhere' is AI model training, which is the process of feeding the algorithm data, looking at the results and then making the appropriate changes to increase its efficiency and accuracy.
Don't miss: Study SG consumers don't think businesses are transparent on their use of AI
There are many types of AI training out there as well as sources of training data for AI models. These include text, image and audio data, sensor data, simulation data and geospatial data to name a few.
Another way that professionals train AI models up is through the use of synthetic data. Synthetic data refers to artificially generated data rather than data collected from real-world sources. This can be very useful for training AI models especially when real data is limited, expensive to obtain, or contains sensitive information.
Synthetic data is gaining popularity as an AI training source because it can offer enhanced safety and privacy as compared to real-world data in certain contexts.
For one, synthetic data can be generated in a way that preserves certain properties of the original data without revealing sensitive information. There is also a reduced risk of data breaches, less liability, more flexibility with data usage and a better chance of compliance with regulations.
With synthetic data gaining popularity, MARKETING-INTERACTIVE spoke to two AI experts to find out how it could be the answer to privacy and accuracy concerns when it comes to the use of AI platforms.
What is synthetic data?
Synthetic data refers to artificially generated data that mimics the characteristics of real-world data.
In contrast to real data, which originates from observations of natural occurrences, synthetic data refers to artificially generated information, which is the outcome of employing advanced algorithms that have been trained on real-world data sets through the power of deep learning, explained Siddharth Jhanji, senior manager (domain leader), data architecture and engineering at Ekimetric.
"Using models to generate data, we can detect patterns, structures, correlations and more within the real data and generate brand-new data with the same pattern," he said.
With new Gen AI text-to-image and text-to-video models coming in such as Mid Journey and Sora, synthetic data can be created to create new images and videos that have similar patterns, he added.
How do you train an AI model on synthetic data?
An AI model can be trained on various types of data, including real data, synthetic data, and hybrid datasets, explained Jhanji.
The choice of data type then depends on the specific application, availability of real data, privacy considerations, and the desired level of control and scalability. He added:
Real data captures the nuances of the real world, while simulated and hybrid datasets offer flexibility and privacy advantages.
Jhanji continued by saying that synthetic data, in particular, provides a powerful tool for overcoming privacy constraints and generating large volumes of data with desired characteristics, although it may not fully capture the complexity and variability of real-world data.
Suppose you want to train a text-to-image model like Midjourney, which generates images based on given textual descriptions, he explained. To train the model, you can use synthetic data by generating artificial textual descriptions and corresponding images.
For instance, you can create descriptions such as 'a red car on a sunny beach' and generate an image that matches this description. This synthetic data helps enhance the model's ability to generate images based on text.
What are the pros and cons of training an AI model on synthetic data?
The pros of training an AI model on synthetic data include its ability to preserve privacy, generate diverse scenarios for training, and reduce bias present in real data, said Milind, an AI Scientist from Mercedes, who was expressing independent views.
However, the cons involve the risk of not fully representing real-world complexities, leading to potential performance limitations in practical applications, he explained.
"Difficulties in using synthetic data include the challenge of accurately capturing the full complexity of real-world data, as well as the need for rigorous validation to ensure its effectiveness," he said, adding:
Synthetic data is not a default due to the inherent limitations in fully replicating the intricacies of genuine data, which can impact the model's performance in real-world settings.
Adding to his point, Jhanji explained that real data provides a more accurate representation of natural occurrences, and the model may struggle to generalise well to unseen real-world situations.
For example, said Jhanji, if you're training a text-to-video model such as Sora, generating synthetic data may not fully capture the intricacies and diversity of real-world videos.
He explained that synthetic videos may lack the complexity, randomness, and nuances found in real footage, making it challenging for the model to learn and generalise effectively.
"These limitations make synthetic data not a default choice, as it may not accurately represent the intricacies of real data and can lead to suboptimal model performance when applied to real-world scenarios," he said, adding that a careful evaluation of the trade-offs between synthetic and real data is essential for effective AI model training.
Copyright issues are of paramount concern to marketers. How can synthetic data be used to mitigate these issues for marketers using AI for campaigns?
Synthetic data can be used to mitigate copyright issues for marketers by providing an alternative to using proprietary or sensitive real data, said Milind.
"By generating synthetic data that closely resembles characteristics of the original without infringing on copyright, marketers can use it for training AI models and conducting campaigns without legal concerns," he said.
Jhanji added to his point by saying that marketers can utilise synthetic data to create realistic consumer profiles, simulate customer behaviour, and generate campaign-related content, reducing the need to rely on copyrighted data or content.
He added that in his opinion, synthetic data is likely to play a significant role in the future due to its potential for privacy preservation, scalability, and cost efficiency.
"However, its adoption and impact will depend on advancements in generating more realistic and representative synthetic data, addressing challenges related to biases and interpretability, and ensuring transparency and trust among users and stakeholders," he said.
Join us this coming 24 - 25 April for #Content360, a two-day extravaganza centered around four core thematic pillars: Explore with AI; Insight-powered strategies; Content as an experience; and Embrace the future. Immerse yourself in learning to curate content with creativity, critical thinking, and confidence with us at Content360!
Related articles:
AI, data, and social commerce: Reshaping SEA's marketing playbook for 2024
Meta to contribute data to the study of well-being
Unleashing the power of AI to elevate the customer experience
share on
Free newsletter
Get the daily lowdown on Asia's top marketing stories.
We break down the big and messy topics of the day so you're updated on the most important developments in Asia's marketing development – for free.
subscribe now open in new window